Kevin M. Keene
Racial and Social Equity in Assessments Part III — Remediation and Correction
If statistical analysis, visualizations and root cause analyses indicate that bias is present, what can be done to address or correct the problem? The answer is dependent on the extent of the indicated bias — systemic or anecdotal — and the cause(s) or source(s) of bias.
It is recommended that the remediation process should start with a careful deconstruction of the entire validation process and all subsystems to identify possible sources of bias. In addition to looking at each subsystem, the system(s) may be employing “faulty logic” which is affecting the entire process.
Here are some examples of “faulty logic” that I have encountered. In each case, see if you can identify the problem. How could each of them create bias in the assessments?
• Assume that the interior condition of a property matches the exterior, or what can be seen from the curb.
• Assume a property to be in average condition unless there is evidence to the contrary.
• Assume a sale to be valid unless there is evidence to the contrary.
• Assume a sale to be invalid unless there is evidence to the contrary.
• If a property has been in below average or worse condition for more than a prescribed period of time, upgrade it to average condition.
• If there is a garage door, assume the number of garage spaces based on the size of the door or the number of doors.
• If there is no central air compressor on the property, code “central air” as “no”.
• Code a flipped property as a “rehab”.
• After a prescribed period of time, downgrade “new construction” or “rehab” to “above average”.
• If a sale has a higher that typical price, assume it is a “rehab”.
• Assume that all properties are moving up or down at the same rate.
• Values in this area are just too low / too high. Reduce / raise them accordingly.
• there is a set of documented standards, and everyone follows them.
Here are some examples of what might be found in examining the various subsystems. These examples will neither be exhaustive or definitive but are useful as guidelines.
Risks in Data Collection and Gathering
Even when assessments are based on objectively observed attributes of properties, using the most demographically agnostic processes available, it is possible for the biases of those who collect and enter the underlying data to introduce bias into the process.
Some attributes that are significant determinants of value are subjective in nature (in the eye of the beholder). Achieving consistency in the application of quality codes, condition codes, and delineation of locations is essential in minimizing inequities in the valuation process.
Some attributes or neighborhoods may not have adequate representation, leading to presumptions of comparability which may be inappropriate.
Let’s consider a common example of how coding bias can produce inequity through the application of codes for the condition of improvements. Condition of Improvements is one of the prime reasons for variance in price in most markets. Condition is best considered in absolute terms, based on the degree of observed deferred maintenance and not related to any other attribute. There should be written objective guidelines for the assignment of each code, so training can be consistent for all data collectors. If condition codes are subject to the interpretation of each data collector, the results will be inconsistent and possibly useless for purposes of valuation.
If a data collector is working in a neighborhood where most of the properties have a lot of deferred maintenance (which should objectively be coded as “below average” or “poor” condition), that person — taking a relative approach — might code those as “average”. In this valuation model, “below average” condition properties should be valued at about 38% less than those in ‘average’ condition.

None of the properties that have been miscoded by the data collector as “average” will get the appropriate downward adjustment from the sales model, resulting in all of them being overvalued when the valuation process is applied! Even if that data collector had no intention of creating inequity, that will be the result for all of the miscoded properties.
This could be a matter of incompetence or lack of training. Or it could be the result of recalcitrance or malfeasance. I was once training a group of data collectors on the application of condition codes. I asked one of the trainees, who I suspected was not following the written objective standards, how they applied Condition Codes to his inventory. Their response was “It depends on how I feel that day.” Not only were they not following the objective department standards, they had no consistent internal standard to which they adhered! To make matters worse, it was clear that they had no intention of changing their behavior based on any instruction they were given, despite the fact that their performance statistics were the worst in the department. Ultimately, they were re-assigned out of the evaluation role.
Some data collection systems will combine multiple attributes into one data element, such as combining condition and quality of construction of improvements into one code. Attribute data should be as granular as possible. Each element should convey one — and only one — piece of information. Let’s say we have a system of seven condition codes and five quality of construction codes. We probably have enough observations of each code to create adjustments for each of them. If we roll them into one attribute, we now have thirty five possible combinations of the two attributes, which may be flattened into a lesser number of codes. Variance can no longer be sourced to a specific attribute, and it is no longer possible to isolate the contributory effect of either attribute on value. The value of the data has become muddy and inconclusive. Condition should tell us about condition. Quality should tell us about construction quality. Review all data elements that are collected and make them as granular as possible.
Problems with data collection typically lead to anecdotal bias and can usually be corrected by re-training data collectors, providing written objective standards for coding, re-collecting the data in question, and adding a layer of quality control to the process.
Risks in Sales Validation
The rules and methods by which sales are selected for use in valuation, or validated, is another common entry point for bias. Sales validation consists of two parts:
• Coding transactions as either valid or invalid as indicators of value for comparable properties and
• Capturing the attributes or characteristics of a property at the time that it was sold.
Sales validation is a subjective process and is especially susceptible to human bias. It is very difficult to create an automated process that can determine if all of the conditions that constitute a valid transaction are present. I have seen some modelers attempt to script sales validation. Upon examination those efforts have been woefully inadequate, or at best a poor substitute for a rigorous process that examines the conditions of every transaction in making a validation decision.
There is value in having sales validation performed by the same people who assign value. Reviewing sales keeps them in contact with changes in the market and provides a framework from which they can they can relate market values. The risk is that each person may have different processes and standards for validating sales, which leads to inconsistent quality of the process as a whole.
In our Philadelphia office, we traditionally favored having each appraiser validate the sales for their inventory. Over time, we found that capture of attributes at time of sale and entry of validation codes was not consistently applied or performed. Some of the issue was addressed through training, but there were those who routinely eliminated high priced sales that would lead to increases in market values, thereby decreasing the number of complaints and appeals the they would receive, as well as those that did the opposite, perhaps feeling that their duty was to generate as much revenue as possible for the city. The why of what they were doing is not important. The problem was the inconsistency of the results. We made a decision to create a small unit that would validate all sales. They were given a prescribed documented process to follow for validating all transactions, and transactions were randomly assigned so that no one person was exclusively validating a specific area or type of property. The result was a greatly improved process with much more consistent results. The performance of our valuation models improved with the input of better quality data. The appraisers had access to the results of the Validation Unit’s work, so they could still understand how the market was changing, but removing them from making the sales validation decisions proved to be a great improvement to the process.
Bias in the sales validation process will lead to either anecdotal or systemic bias in assessments to the degree of influence of each validator, or to the validators as a whole, if training is the issue.
Risks in Modeling
I was once asked if regression models can be biased. Models are statistical generalizations, and ALL generalizations contain bias. Sometimes the bias is in the data used for the model. At other times, it is the assumptions that are made when there is not enough data for a specific attribute. We hope that models are biased in ways that support representation of the local market dynamics, but it is possible that they may prevent or hamper such representation.
Models can be built in such a way that the code itself contains bias. Transformation is the art of preparing raw data for use in a model. For example, there are many ways to transform building square footage for use in a model. The transformation that is selected can be biased in favor of smaller or larger dwellings. Categorical transformations can group neighborhoods that have few or no sales with other neighborhoods that might not actually be comparable. Mixing markets within one model can distort the results for all cases.
Models should not be built to produce an expected result. If a modeler has an expectation of how the model should turn out, it is not difficult to create the expected result. A modeler once told me that before they built the model, they knew how much each attribute affected price. So much for objectively letting the data tell the story! Selective inclusion/exclusion of observations; constraining variables or coefficients; inappropriate selection of attributes; even selection of the form of the model can “direct” the outcome so that confirmation bias becomes a serious issue. Examine a significant sample of model code. What are the untested assumptions that are being made? Are the biases of the modeler(s) influencing the outcome?
Avoid use of demographic attributes in valuation models. Attributes like crime, levels of educational attainment, proximity to certain religious institutions, and income levels should not be used.
Bias in modeling can produce widespread or systemic bias in assessments. This can best be corrected by re-writing the models, removing biased code, and replacing transformations that create bias.
Risks in Location Definition
Neighborhoods should be areas within which properties with similar characteristics will sell for similar prices. It is not necessary for all properties within a neighborhood to be similar. Neighborhoods should be created that do not consider who lives in or owns the properties, and should be frequently updated to reflect changes in the market. Location variables or definitions should not be correlated with race, income, religion or any other demographics.
Zip codes and census tracts are created based on demographics. Many municipalities use them to define location. If they are used to define neighborhoods, social nd racial bias are inherent in the valuation process.
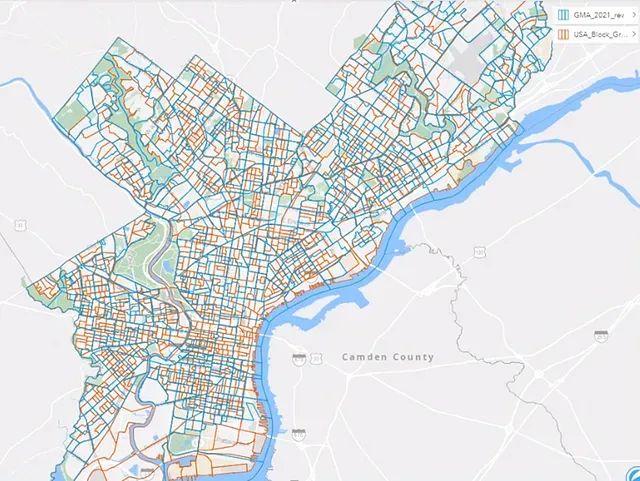
This map shows mass appraisal location boundaries in blue and census block group boundaries in red. The boundaries do not align, demonstrating that mass appraisal boundaries are not demographically correlated in this system.
Correlation of mass appraisal location boundaries with demographic based boundaries will often result in systemic bias, and can best be addressed by changing the method of creating location definitions or replacing fixed locations with geographic weighted regression or using a spatial model.
Risk in Review and Oversight
The valuation process produces estimated values for properties. Even the best methods are subject to significant rates of error, so some sort of process to review projected values is needed. The review process includes revaluation projections prior to formalization and release to the public and both informal and formal appeals. Reviewing values produced by the valuation process is a common entry point for human bias. If multiple reviewers are working without following common guidelines and procedures, the body of review work may be inconsistent and can easily reflect the bias of the reviewer(s).
When deconstructing the review process, one should ask:
How are records selected for review? Is it possible to review groups of related records at one time, or is the review conducted one account at a time?
Is it possible to make decisions about groups of related accounts? How are changes processed and applied? When review or decision making is accomplished on a one-property-at-a-time basis, it is difficult to establish or maintain consistent decisions and presents an entry point for bias.
Some reviewers will reduce values or raise values on review for a variety of reasons. Is there at least one level of oversight and approval required, or does the reviewer have full discretion in making changes? Have different reviewers describe their decision-making process.
This issue most often results in anecdotal bias, which can often be addressed with training and provision of consistent oversight.
Political Interference — The Automatic Loser
Many assessment offices exist as part of a political organization. Assessors are not always free to follow the best objective standards and practices.
Overt political interference often takes the form of an official(s) directing values for their constituents be given special consideration. However, political intervention or interference is not necessarily ill intentioned or designed to produce favorable outcomes for a select group of people. Undesirable outcomes are often the unintended consequences of other decisions, such as targeted abatements or exemptions enacted to spur activity or protect a vulnerable population; opportunity zones that convey special considerations; or other directives that tend to affect the market.
If analysis demonstrates that there are other forces in play, the bias argument is lost by definition. Foreknowledge that intervention is discoverable, and that uniformity applies to everyone without favor, can help prevent or even eliminate the presence of political intervention. Political interference will almost always produce systemic bias, and there are no technical remedies. The task becomes educating those who would interfere in the process about the obvious detrimental effects and discouraging such practice.
What are the Remedies?
What should you do if allegations of assessment bias are leveled at your municipality? The first thing is to ensure that the perception of bias is based in fact, not a narrative that is the result of flawed conclusions based on flawed data. Review both the data and the resulting analysis. If possible, engage the analysts to discuss flaws in data and methodology.
Be Proactive — Not Reactive!
First and foremost, adopt a proactive posture. Don’t wait for the story to be told by someone else! Take control of the story. Tell everyone what you have found and what you plan to do. Bias in assessments doesn’t rise overnight and won’t be fixed overnight. It may require several incremental steps, spanning multiple valuation cycles, to correct the problem. Systemic bias is typically the result of a legacy of many years of practice. Anecdotal bias is easier to identify and quicker to correct. It is strongly recommended that allegations of bias be met by demonstrating that qualified analysis has been performed and that plans are in process to remediate bias.
In summary, here is a sixteen-point plan for conducting qualified analysis, reporting discoveries and remediating bias in assessments.
• Practice transparency — be prepared to share data as well as findings.
• Share data files with independent analysts and auditors.
• Use appropriate data when conducting ratio studies and create effective measures for unsold or unrepresented properties.
• Commission a neutral 3rd party to run the analysis. Running the analysis yourself is a viable option if you proactively want to determine the state of equity of your assessments and no report or remediation plan will be published. Once allegations of bias are made, engagement of a third party will be required. In addition, avoidance of integrating assessment data with demographic data might make it a good idea to engage a third party to run the analysis, even if no allegation of bias has been made.
• Report findings to all stakeholders, including the public.
• Re-train staff to collect and manage data more objectively.
• Deconstruct subsystems of the valuation process to identify and eliminate sources of systemic bias.
• Revise the way that data is coded and stored in the CAMA system.
• Invest in improving sales validation.
• Avoid use of demographic attributes in the valuation process.
• Redefine neighborhoods to be racially and socially agnostic.
• Conduct a full revaluation.
• If a full revaluation is not possible, conduct a targeted revaluation.
• Eliminate or limit external interference in the valuation process.
• Create a comprehensive plan to limit or eliminate bias on an ongoing basis.
• Run follow up studies to document progress.
Another option (not recommended!) is to wait for a court order in the wake of allegations of bias. Remember, the Assessor’s office, while ultimately accountable, is typically not a “bad actor”, and is in the best position to identify and correct the bias by actually improving equity. Courts, on the other hand, will tend to impose equality, not equity, which is not the desired outcome.
I hope the information presented in this article is helpful to practitioners across the country, and that I have encouraged colleagues in our industry to think about the bias problem in ways not previously considered. I look forward to the evolution of discussion of this topic, and to further engagement in that discussion.
About the Author
Kevin Keene served the City of Philadelphia for over 30 years as a Real Property Evaluator; Mass Appraisal Analyst; Real Property Supervisor; GIS Manager; Modeling Director and Director of Mass Appraisal and Analysis. He personally designed and directed over sixteen major revaluation projects. He has trained appraisers, modelers and analysts, and has been a frequent presenter at IAAO and URISA conferences.
In his semi-retirement he is the principal at Keene Mass Appraisal Consulting (keenemac.com), offering a variety of Mass Appraisal and educational services. His primary passions are promoting assessment equity, community activism and enjoying life with a loving wife and two adorable dogs.
267.307.6071
Kevin@keenemac.com